Making the Case for Safety of Machine Learning applied to Automated Driving
Simon Burton
Chief Expert Safety Reliability and Availability, Bosch
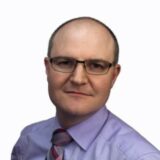
Machine learning technologies such as neural networks show great potential for enabling automated driving functions in an open world context. However, these technologies can only be released for series production if it can be demonstrated to be sufficiently safe. As a result, convincing arguments need to be made for the safety of automated driving systems based on such technologies. This talk examines the various forms in which machine learning can be applied to automated driving and the resulting functional safety challenges. A systems engineering approach is proposed to derive a precise definition of the performance requirements on the function to be implemented on which to base the safety case. A systematic approach to structuring the safety case is introduced and a number of open research questions are presented including a discussion on how to relate machine learning specific performance metrics to system level safety requirements.